Generative Artificial Intelligence (AI) stands at the forefront of innovation, creating novel content, designs, and solutions. As the demands for adaptive and cutting-edge generative AI systems grow, so does the need for methodologies that can navigate the dynamic and iterative nature of these projects. In this blog post, we’ll delve into how Agile methodologies prove to be a strategic ally in the realm of Generative AI, facilitating creativity, collaboration, and rapid adaptation.
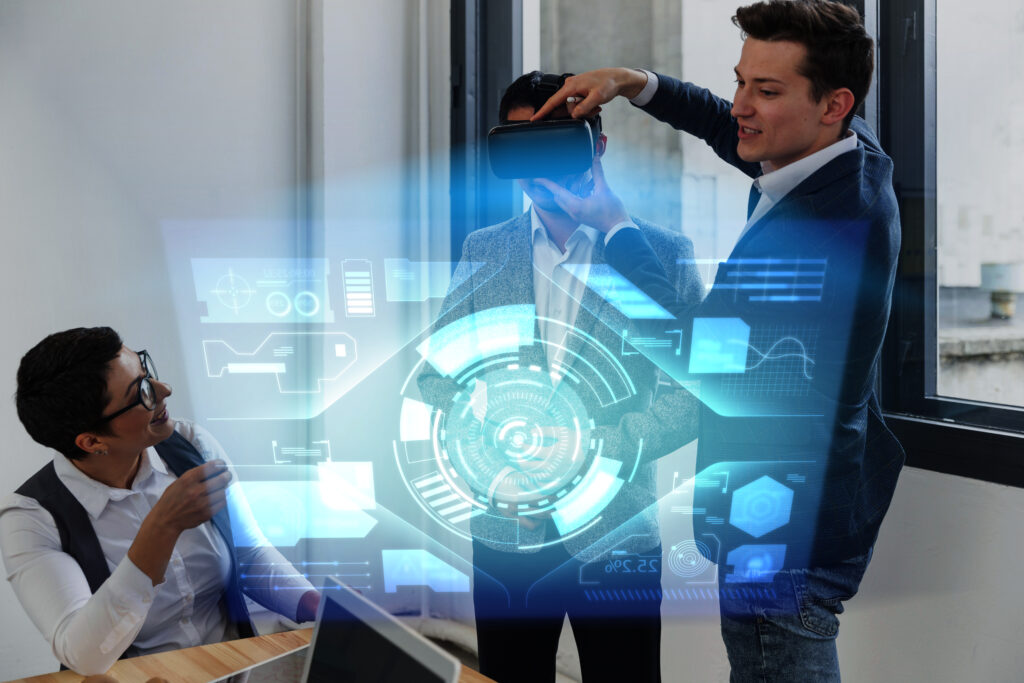
- Iterative Progress in Generative AI:
Generative AI projects often involve the creation of models that evolve through multiple iterations. Agile’s iterative approach aligns seamlessly with this process. By breaking down the generative AI development into manageable increments, teams can regularly deliver tangible outputs, allowing stakeholders to witness and provide feedback on the evolving creativity of the system. - Continuous Collaboration and Cross-Functional Teams:
The creative potential of Generative AI flourishes in an environment that encourages collaboration between diverse skill sets. Agile’s emphasis on cross-functional teams, comprising data scientists, domain experts, and creatives, fosters an atmosphere where different perspectives converge to fuel innovation. This collaborative synergy enhances the generative AI process by integrating varied insights and expertise. - Customer-Centric Creativity:
Generative AI often serves creative industries, and understanding user preferences is paramount. Agile’s customer-centric approach, emphasizing frequent user feedback, is instrumental in refining generative models. Through regular showcases of generated content, stakeholders can provide feedback that guides the AI towards producing outputs that align more closely with user expectations. - Flexibility and Adaptability:
The landscape of Generative AI is dynamic, with rapidly advancing techniques and shifting creative trends. Agile’s inherent adaptability allows teams to respond promptly to changes in project requirements, incorporate new artistic styles, or adjust the generative algorithms as needed. This flexibility ensures that generative AI systems remain at the forefront of creative expression. - Rapid Prototyping and Experimentation:
Agile methodologies encourage the creation of minimum viable products (MVPs) early in the development process. This approach is particularly beneficial in Generative AI, where rapid prototyping and experimentation can unveil unforeseen possibilities and inspire further creativity. It also allows teams to gauge the viability and potential impact of different generative approaches. - Balancing Innovation with Quality:
While speed and innovation are crucial in Generative AI, maintaining the quality of generated content is equally important. Agile’s focus on sustainable development and regular testing ensures that the creative outputs meet not only the speed requirements but also the quality standards expected by users.
Conclusion
The fusion of Agile methodologies with Generative AI forms a strategic partnership that elevates creative innovation to unprecedented levels. Embracing agility enables teams to adeptly navigate the iterative and dynamic generative AI landscape, fostering collaboration, embracing change, and producing creative outputs that deeply connect with users. In the constantly evolving realm of AI, characterized by boundless creativity, adopting an Agile approach is not merely advantageous but imperative for fully unleashing the potential of generative systems.